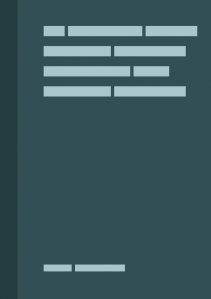
Research methods
We continue to make advances in developing models and methods to study the dynamic behaviour of individuals and firms, the structure of the education, labour and marriage markets, and their implications for policy design and evaluation.
Focus on
Showing 81 – 100 of 1019 results
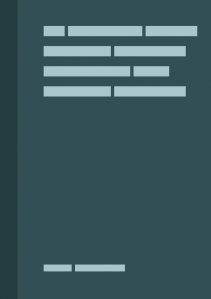
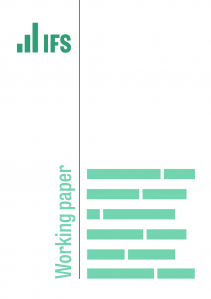
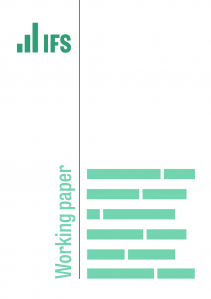
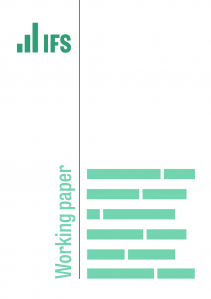
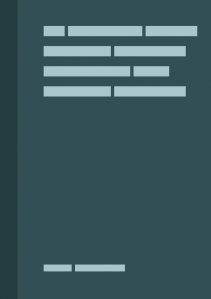
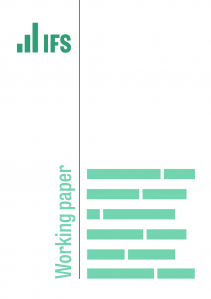
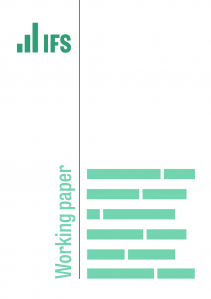
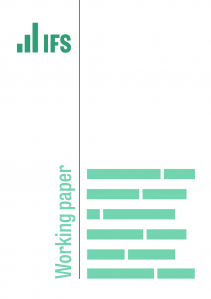
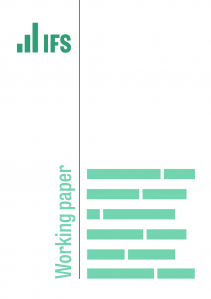
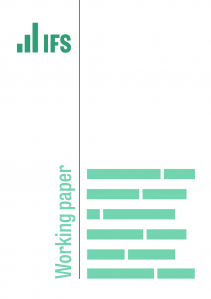
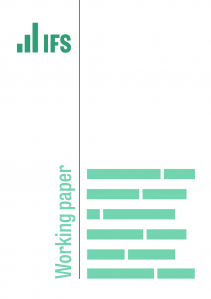
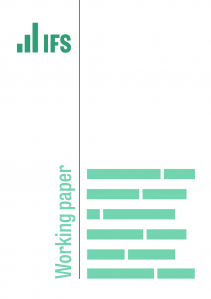
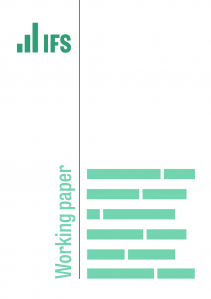
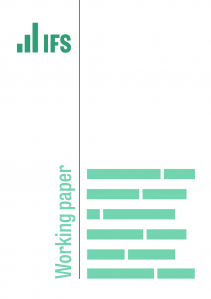
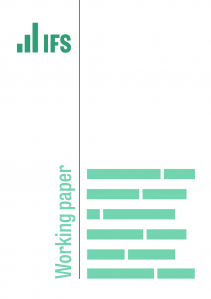
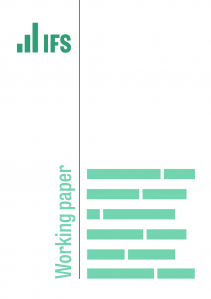
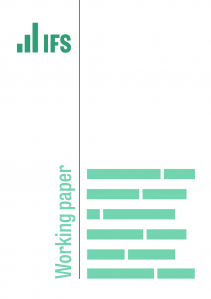
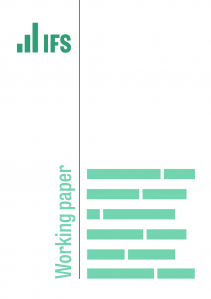
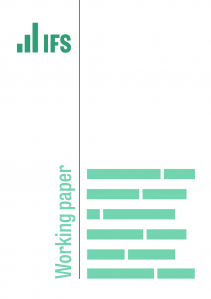
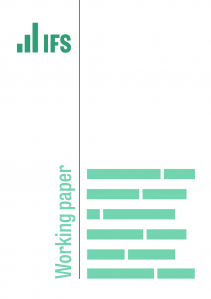